Biomarker discovery and beyond for diagnosis of bladder diseases
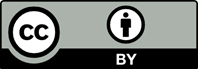
Molecular biosignatures of altered cellular landscapes and functions have been casually linked with pathological conditions, which imply the promise of biomarkers specific to bladder diseases, such as bladder cancer and other dysfunctions. Urinary biomarkers are particularly attractive due to costs, time, and the minimal and noninvasive efforts acquiring urine. The evolution of omics platforms and bioinformatics for analyzing the genome, epigenome, transcriptome, proteome, lipidome, metabolome, etc., have enabled us to develop more sensitive and disease-specific biomarkers. These discoveries broaden our understanding of the complex biology and pathophysiology of bladder diseases, which can ultimately be translated into the clinical setting. In this short review, we will discuss current efforts on identification of promising urinary biomarkers of bladder diseases and their roles in diagnosis and monitoring. With these considerations, we also aim to provide a prospective view of how we can further utilize these bladder biomarkers in developing ideal and smart medical devices that would be applied in the clinic.
Urine and urinary biomarkers for bladder diseases
Multi-omics application for bladder biomarker development
Microbiome studies in urological diseases
Challenges and consideration in urine biomarker development
Biosensor for the detection of urinary biomarkers
Digital applications of biosensors
Concluding remarks
-
-
-
Coppens A, Speeckaert M, Delanghe J (2010) The pre-analytical challenges of routine urinalysis. Acta Clin Belg 65: 182-189.doi: https://doi.org/10.1179/acb.2010.038. [View Article] [PubMed] [Google Scholar]
-
Howanitz PJ, Howanitz JH (1983) Quality control for the clinical laboratory. Clin Lab Med 3: 541-551.doi: https://doi.org/10.1016/S0272-2712(18)30974-0. [View Article] [PubMed] [Google Scholar]
-
Delanghe J, Speeckaert M (2014) Preanalytical requirements of urinalysis. Biochem Med (Zagreb) 24: 89-104.doi: https://doi.org/10.11613/BM.2014.011. [View Article] [PubMed] [Google Scholar]
-
Fogazzi GB, Verdesca S, Garigali G (2008) Urinalysis: core curriculum 2008. Am J Kidney Dis 51: 1052-1067.doi: https://doi.org/10.1053/j.ajkd.2007.11.039. [View Article] [PubMed] [Google Scholar]
-
Bouatra S, Aziat F, Mandal R, Guo AC, Wilson MR, et al. (2013) The human urine metabolome. PLoS One 8: e73076.doi: https://doi.org/10.1371/journal.pone.0073076. [View Article] [PubMed] [Google Scholar]
-
Hg DS, Siltberg-Liberles J (2016) Paralog-specific patterns of structural disorder and phosphorylation in the vertebrate SH3-SH2-tyrosine kinase protein family. Genome Biol Evol 8: 2806-2825.doi: https://doi.org/10.1093/gbe/evw194. [View Article] [PubMed] [Google Scholar]
-
Glorennec P, Serrano T, Fravallo M, Warembourg C, Monfort C, et al. (2017) Determinants of children's exposure to pyrethroid insecticides in western France. Environ Int 104: 76-82.doi: https://doi.org/10.1016/j.envint.2017.04.007. [View Article] [PubMed] [Google Scholar]
-
Filip S, Zoidakis J, Vlahou A, Mischak H (2014) Advances in urinary proteome analysis and applications in systems biology. Bioanalysis 6: 2549-2469.doi: https://doi.org/10.4155/bio.14.210. [View Article] [PubMed] [Google Scholar]
-
Apodaca G, Balestreire E, Birder LA (2007) The uroepithelial-associated sensory web. Kidney Int 72: 1057-1064.doi: https://doi.org/10.1038/sj.ki.5002439. [View Article] [PubMed] [Google Scholar]
-
Xing J, Reynolds JP (2018) Diagnostic advances in urine cytology. Surg Pathol Clin 11: 601-610.doi: https://doi.org/10.1016/j.path.2018.06.001. [View Article] [PubMed] [Google Scholar]
-
Nadkarni GN, Coca SG, Meisner A, Patel S, Kerr KF, et al. (2017) Urinalysis findings and urinary kidney injury biomarker concentrations. BMC Nephrol 18: 218.doi: https://doi.org/10.1186/s12882-017-0629-z. [View Article] [PubMed] [Google Scholar]
-
Rhee EP (2018) How omics data can be used in nephrology. Am J Kidney Dis 72: 129-135.doi: https://doi.org/10.1053/j.ajkd.2017.12.008. [View Article] [PubMed] [Google Scholar]
-
Miyake M, Owari T, Hori S, Fujimoto K (2019) Significant lack of urine-based biomarkers to replace cystoscopy for the surveillance of non-muscle invasive bladder cancer. Transl Androl Urol 8(Suppl 3): S332-S334.doi: https://doi.org/10.21037/tau.2019.05.07. [View Article] [PubMed] [Google Scholar]
-
Zhao M, Li M, Yang Y, Guo Z, Sun Y, et al. (2017) A comprehensive analysis and annotation of human normal urinary proteome. Sci Rep 7: 3024.doi: https://doi.org/10.1038/s41598-017-03226-6. [View Article] [PubMed] [Google Scholar]
-
Salomonis N (2018) Integrative analysis of proteomics data to obtain clinically relevant markers. Methods Mol Biol 1788: 89-111.doi: https://doi.org/10.1007/7651_2017_94. [View Article] [PubMed] [Google Scholar]
-
Zhang H, Fan Y, Xia L, Gao C, Tong X, et al. (2017) The impact of advanced proteomics in the search for markers and therapeutic targets of bladder cancer. Tumour Biol 39: 1010428317691183.doi: https://doi.org/10.1177/1010428317691183. [View Article] [PubMed] [Google Scholar]
-
Onile OS, Calder B, Soares NC, Anumudu CI, Blackburn JM (2017) Quantitative label-free proteomic analysis of human urine to identify novel candidate protein biomarkers for schistosomiasis. PLoS Negl Trop Dis 11: e0006045.doi: https://doi.org/10.1371/journal.pntd.0006045. [View Article] [PubMed] [Google Scholar]
-
Adebayo AS, Mundhe SD, Awobode HO, Onile OS, Agunloye AM, et al. (2018) Metabolite profiling for biomarkers in Schistosoma haematobium infection and associated bladder pathologies. PLoS Negl Trop Dis 12: e0006452.doi: https://doi.org/10.1371/journal.pntd.0006452. [View Article] [PubMed] [Google Scholar]
-
Zhong X, Isharwal S, Naples JM, Shiff C, Veltri RW, et al. (2013) Hypermethylation of genes detected in urine from Ghanaian adults with bladder pathology associated with Schistosoma haematobium infection. PLoS One 8: e59089.doi: https://doi.org/10.1371/journal.pone.0059089. [View Article] [PubMed] [Google Scholar]
-
Mitsui T, Kira S, Ihara T, Sawada N, Nakagomi H, et al. (2018) Metabolomics approach to male lower urinary tract symptoms: identification of possible biomarkers and potential targets for new treatments. J Urol 199: 1312-1318.doi: https://doi.org/10.1016/j.juro.2017.11.070. [View Article] [PubMed] [Google Scholar]
-
De Palma G, Di Lorenzo VF, Krol S, Paradiso AV (2019) Urinary exosomal shuttle RNA: Promising cancer diagnosis biomarkers of lower urinary tract. Int J Biol Markers 34: 101-107.doi: https://doi.org/10.1177/1724600819827023. [View Article] [PubMed] [Google Scholar]
-
Junker K, Heinzelmann J, Beckham C, Ochiya T, Jenster G (2016) Extracellular vesicles and their role in urologic malignancies. Eur Urol 70: 323-331.doi: https://doi.org/10.1016/j.eururo.2016.02.046. [View Article] [PubMed] [Google Scholar]
-
Schwabe RF, Jobin C (2013) The microbiome and cancer. Nat Rev Cancer 13: 800-812.doi: https://doi.org/10.1038/nrc3610. [View Article] [PubMed] [Google Scholar]
-
Xu W, Yang L, Lee P, Huang WC, Nossa C, et al. (2014) Mini-review: perspective of the microbiome in the pathogenesis of urothelial carcinoma. Am J Clin Exp Urol 2: 57-61. [PubMed] [Google Scholar]
-
Alfano M, Canducci F, Nebuloni M, Clementi M, Montorsi F, et al. (2016) The interplay of extracellular matrix and microbiome in urothelial bladder cancer. Nat Rev Urol 13: 77-90.doi: https://doi.org/10.1038/nrurol.2015.292. [View Article] [PubMed] [Google Scholar]
-
Wu P, Zhang G, Zhao J, Chen J, Chen Y, et al. (2018) Profiling the urinary microbiota in male patients with bladder cancer in China. Front Cell Infect Microbiol 8: 167.doi: https://doi.org/10.3389/fcimb.2018.00167. [View Article] [PubMed] [Google Scholar]
-
Markowski MC, Boorjian SA, Burton JP, Hahn NM, Ingersoll MA, et al. (2019) The microbiome and genitourinary cancer: a collaborative review. Eur Urol 75: 637-646.doi: https://doi.org/10.1016/j.eururo.2018.12.043. [View Article] [PubMed] [Google Scholar]
-
Wroblewski LE, Peek RM (2010) Wilson KT. Helicobacter pylori and gastric cancer: factors that modulate disease risk. Clin Microbiol Rev 23: 713-739.doi: https://doi.org/10.1128/CMR.00011-10. [View Article] [PubMed] [Google Scholar]
-
Dahmus JD, Kotler DL, Kastenberg DM, Kistler CA (2018) The gut microbiome and colorectal cancer: a review of bacterial pathogenesis. J Gastrointest Oncol 9: 769-777.doi: https://doi.org/10.21037/jgo.2018.04.07. [View Article] [PubMed] [Google Scholar]
-
Spanogiannopoulos P, Bess EN, Carmody RN, Turnbaugh PJ (2016) The microbial pharmacists within us: a metagenomic view of xenobiotic metabolism. Nat Rev Microbiol 14: 273-287.doi: https://doi.org/10.1038/nrmicro.2016.17. [View Article] [PubMed] [Google Scholar]
-
Geller LT, Barzily-Rokni M, Danino T, Jonas OH, Shental N, et al. (2017) Potential role of intratumor bacteria in mediating tumor resistance to the chemotherapeutic drug gemcitabine. Science 357: 1156-1160.doi: https://doi.org/10.1126/science.aah5043. [View Article] [PubMed] [Google Scholar]
-
Wallace BD, Wang H, Lane KT, Scott JE, Orans J, et al. (2010) Alleviating cancer drug toxicity by inhibiting a bacterial enzyme. Science 330: 831-835.doi: https://doi.org/10.1126/science.1191175. [View Article] [PubMed] [Google Scholar]
-
Domenici L, Monti M, Bracchi C, Giorgini M, Colagiovanni V, et al. (2016) D-mannose: a promising support for acute urinary tract infections in women. A pilot study. Eur Rev Med Pharmacol Sci 20: 2920-2925. [PubMed] [Google Scholar]
-
Bucevic Popovic V, Situm M, Chow CT, Chan LS, Roje B, et al. (2018) The urinary microbiome associated with bladder cancer. Sci Rep 8: 12157.doi: https://doi.org/10.1038/s41598-018-29054-w. [View Article] [PubMed] [Google Scholar]
-
Nickel JC, Stephens-Shields AJ, Landis JR, Mullins C, Bokhoven, A. van , et al. (2019) A culture-independent analysis of the microbiota of female interstitial cystitis/bladder pain syndrome participants in the MAPP research network. J Clin Med 8.doi: https://doi.org/10.3390/jcm8030415. [View Article] [PubMed] [Google Scholar]
-
Govender Y, Gabriel I, Minassian V, Fichorova R (2019) The Current Evidence on the Association Between the Urinary Microbiome and Urinary Incontinence in Women. Front Cell Infect Microbiol 9: 133.doi: https://doi.org/10.3389/fcimb.2019.00133. [View Article] [PubMed] [Google Scholar]
-
Bresler L, Price TK, Hilt EE, Joyce C, Fitzgerald CM, et al. (2019) Female lower urinary tract microbiota do not associate with IC/PBS symptoms: a case-controlled study. Int Urogynecol J 30: 1835-1842.doi: https://doi.org/10.1007/s00192-019-03942-9. [View Article] [PubMed] [Google Scholar]
-
Greenberg JH, Parikh CR (2017) Biomarkers for diagnosis and prognosis of AKI in children: one size does not fit all. Clin J Am Soc Nephrol 12: 1551-1557.doi: https://doi.org/10.2215/CJN.12851216. [View Article] [PubMed] [Google Scholar]
-
Psihogios NG, Gazi IF, Elisaf MS, Seferiadis KI, Bairaktari ET (2008) Gender-related and age-related urinalysis of healthy subjects by NMR-based metabonomics. NMR Biomed 21: 195-207.doi: https://doi.org/10.1002/nbm.1176. [View Article] [PubMed] [Google Scholar]
-
Guo Z, Zhang Y, Zou L, Wang D, Shao C, et al. (2015) A proteomic analysis of individual and gender variations in normal human urine and cerebrospinal fluid using iTRAQ quantification. PLoS One 10: e0133270.doi: https://doi.org/10.1371/journal.pone.0133270. [View Article] [PubMed] [Google Scholar]
-
Chen Z, Kim J (2016) Urinary proteomics and metabolomics studies to monitor bladder health and urological diseases. BMC Urol 16: 11.doi: https://doi.org/10.1186/s12894-016-0129-7. [View Article] [PubMed] [Google Scholar]
-
Antunes-Lopes T, Coelho A, Pinto R, Barros SC, Cruz CD, et al. (2016) Urinary neurotrophin levels increase in women with stress urinary incontinence after a midurethral sling procedure. Urology 99: 49-56.doi: https://doi.org/10.1016/j.urology.2016.08.048. [View Article] [PubMed] [Google Scholar]
-
Kim J, Campbell AS, de Ávila BE, Wang J (2019) Wearable biosensors for healthcare monitoring. Nat Biotechnol 37: 389-406.doi: https://doi.org/10.1038/s41587-019-0045-y. [View Article] [PubMed] [Google Scholar]
-
McKeating KS, Aube A, Masson JF (2016) Biosensors and nanobiosensors for therapeutic drug and response monitoring. Analyst 141: 429-449.doi: https://doi.org/10.1039/c5an01861g. [View Article] [PubMed] [Google Scholar]
-
Liu X, Lillehoj PB (2017) Embroidered electrochemical sensors on gauze for rapid quantification of wound biomarkers. Biosens Bioelectron 98: 189-194.doi: https://doi.org/10.1016/j.bios.2017.06.053. [View Article] [PubMed] [Google Scholar]
-
Cho S, Park TS, Nahapetian TG, Yoon JY (2015) Smartphone-based, sensitive microPAD detection of urinary tract infection and gonorrhea. Biosens Bioelectron 74: 601-611.doi: https://doi.org/10.1016/j.bios.2015.07.014. [View Article] [PubMed] [Google Scholar]
-
Noiphung J, Laiwattanapaisal W (2019) Multifunctional paper-based analytical device for in situ cultivation and screening of Escherichia coli infections. Sci Rep 9: 1555.doi: https://doi.org/10.1038/s41598-018-38159-1. [View Article] [PubMed] [Google Scholar]
-
Chuang CH, Yc, Du , Wu TF, Chen CH, Lee DH, et al. (2016) Immunosensor for the ultrasensitive and quantitative detection of bladder cancer in point of care testing. Biosens Bioelectron 84: 126-132.doi: https://doi.org/10.1016/j.bios.2015.12.103. [View Article] [PubMed] [Google Scholar]
-
Smittenaar P, Walker AK, McGill S, Kartsonaki C, Robinson-Vyas RJ, et al. (2018) Harnessing citizen science through mobile phone technology to screen for immunohistochemical biomarkers in bladder cancer. Br J Cancer 119: 220-9.doi: https://doi.org/10.1038/s41416-018-0156-0. [View Article] [PubMed] [Google Scholar]
-
Shan R, Sarkar S, Martin SS (2019) Digital health technology and mobile devices for the management of diabetes mellitus: state of the art. Diabetologia 62: 877-87.doi: https://doi.org/10.1007/s00125-019-4864-7. [View Article] [PubMed] [Google Scholar]
-
Charpentier G, Benhamou PY, Dardari D, Clergeot A, Franc S, et al. (2011) The Diabeo software enabling individualized insulin dose adjustments combined with telemedicine support improves HbA1c in poorly controlled type 1 diabetic patients: a 6-month, randomized, open-label, parallel-group, multicenter trial (TeleDiab 1 Study). Diabetes Care 34: 533-539.doi: https://doi.org/10.2337/dc10-1259. [View Article] [PubMed] [Google Scholar]
-
Rossi MC, Nicolucci A, Lucisano G, Pellegrini F, Di Bartolo P, et al. (2013) Impact of the "Diabetes Interactive Diary" telemedicine system on metabolic control, risk of hypoglycemia, and quality of life: a randomized clinical trial in type 1 diabetes. Diabetes Technol Ther 15: 670-679.doi: https://doi.org/10.1089/dia.2013.0021. [View Article] [PubMed] [Google Scholar]
-
Fernández-Caramés TM, Froiz-Míguez I, Blanco-Novoa O, Fraga-Lamas P (2019) Enabling the internet of mobile crowdsourcing health things: A mobile fog computing, blockchain and IoT based continuous glucose monitoring system for diabetes mellitus research and care. Sensors (Basel) 19:doi: https://doi.org/10.3390/s19153319. [View Article] [PubMed] [Google Scholar]
-
Jain SH, Powers BW, Hawkins JB, Brownstein JS (2015) The digital phenotype. Nat Biotechnol 33: 462-3.doi: https://doi.org/10.1038/nbt.3223. [View Article] [PubMed] [Google Scholar]
-
-